Software companies are increasingly leveraging artificial intelligence (AI) and machine learning (ML) techniques to enhance their products in various ways. Here are some common methods:
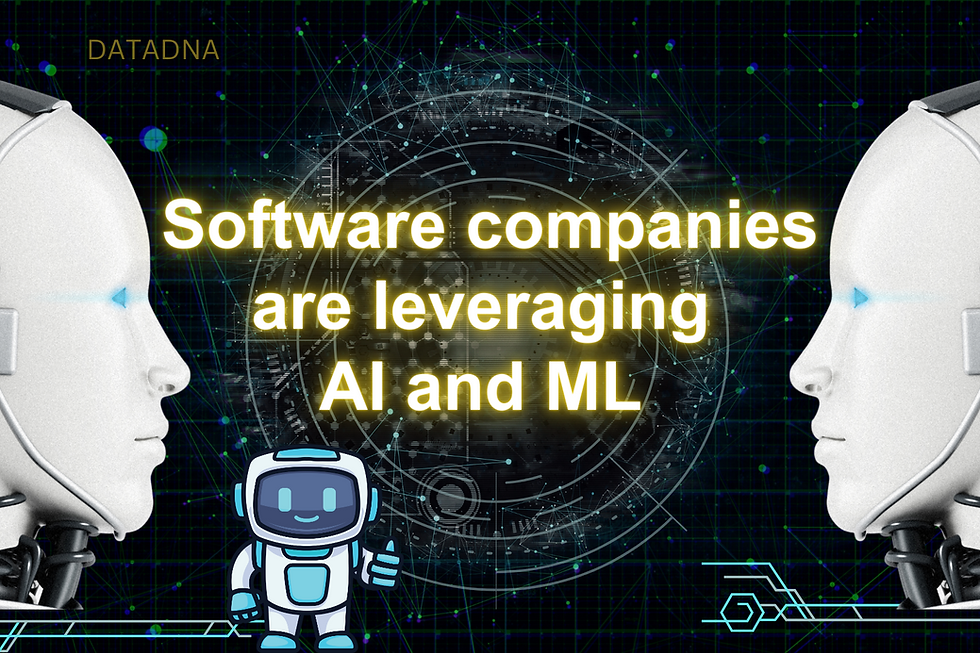
Personalization: AI and ML algorithms can analyze user behavior and preferences to personalize the user experience. For example, recommendation engines in streaming services or e-commerce platforms use ML to suggest content or products based on past interactions.
Predictive Analytics: Software products can utilize ML models to predict user behavior or outcomes. This could include predicting equipment failures in industrial software, forecasting sales trends in retail software, or identifying potential security threats in cybersecurity software.
Automation: AI-powered automation can streamline processes and improve efficiency in software products. For instance, robotic process automation (RPA) can be used to automate repetitive tasks in business software, freeing up human resources for more strategic activities.
Natural Language Processing (NLP): NLP capabilities enable software products to understand and generate human language. Chatbots and virtual assistants are examples of software products that leverage NLP to interact with users in natural language, providing support or performing tasks.
Image and Video Analysis: Software companies are integrating AI and ML algorithms for image and video analysis tasks. This includes applications such as facial recognition in security software, object detection in autonomous vehicles, or content moderation in social media platforms.
Anomaly Detection: ML models can detect anomalies or outliers in data, which is useful for detecting fraudulent activities in financial software, identifying potential security breaches in network monitoring tools, or spotting irregularities in manufacturing processes.
Optimization: AI algorithms can optimize various aspects of software products, such as resource allocation, scheduling, or routing. This is particularly useful in logistics software for route optimization, in resource management software for workload distribution, or in energy management software for optimizing energy consumption.
Quality Assurance: ML techniques can improve the quality assurance process by identifying bugs, anomalies, or performance issues in software products. Automated testing frameworks powered by ML can perform regression testing, identify edge cases, and prioritize test cases based on risk.
Sentiment Analysis: Software products can analyze text data from sources like customer reviews, social media, or surveys to gauge sentiment and feedback. This information can be valuable for product managers to understand customer satisfaction, identify areas for improvement, or monitor brand reputation.
Continuous Improvement: AI and ML models can continuously learn from new data and user feedback, allowing software products to adapt and improve over time. This iterative approach to product enhancement enables companies to stay competitive and meet evolving user needs.
Overall, the integration of AI and ML technologies enables software companies to deliver more intelligent, efficient, and user-centric products that provide value to customers across various industries.
Comments